How artificial intelligence can help us understand the Earth better
In the field of climate and environmental science, we model highly complex systems and manage vast amounts of data. Artificial intelligence can benefit researchers and help us gain a better understanding of the Earth. But as with all technologies, there are risks involved. How should we be using AI?
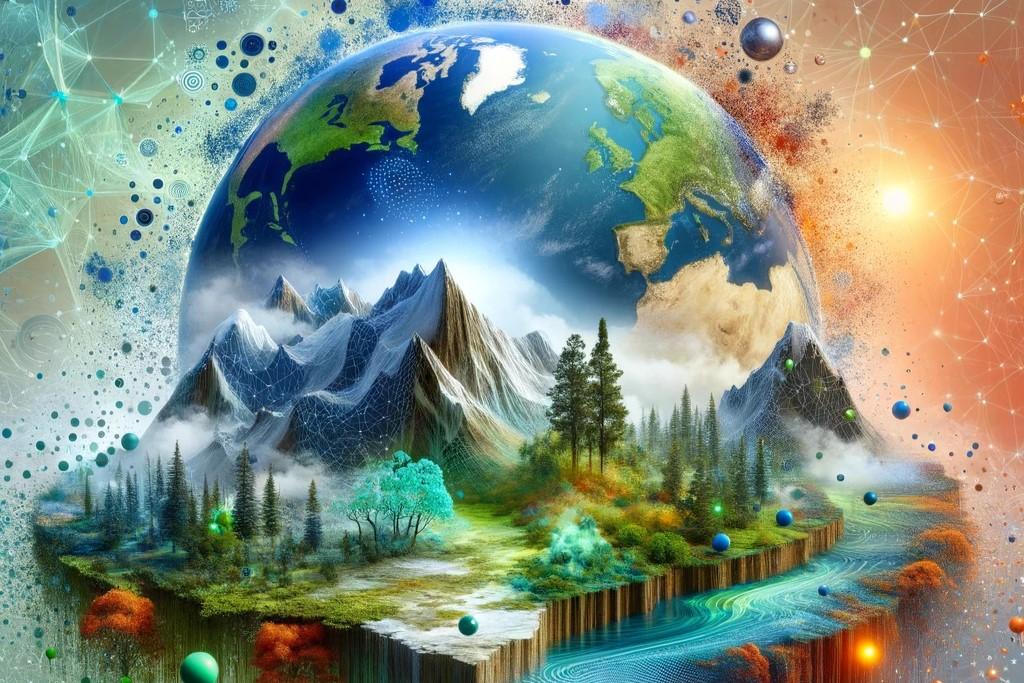
Artificial intelligence (AI) has become a buzzword in the past few years. In particular, generative models such as ChatGPT and Stable Diffusion have gained tremendous attention recently. It has never been easier to create “art” (the question of whether AI-generated art can be considered “real” art is a topic of ongoing debate), write essays or answer emails.
Artificial intelligence made its way into applied research quite a few years ago and many researchers have already been using machine learning methods, a subfield of AI, without even knowing it. The field of AI and machine learning is vast and continuously advancing, with numerous applications in environmental and climate science. These applications range from forecasting of time series or spatial fields, reconstruction of historical observations, improving model output or pre-processing data to assisting with programming or summarising research articles.
Looking forward in time
Most of the time, we are interested in the future: tomorrow’s weather or the climate a thousand years from now. Traditionally, we relied on numerical models to predict the weather and climate. Numerical models are based on basic physical principles and are able to produce accurate results – most of the time. However, running them is often very expensive and slow. In recent years, it has been shown that AI models can perform as well as – or better than – the classical models.
For example, generative models have demonstrated the ability to realistically predict precipitation fields and even outperform classical weather models. Echo state networks (a type of neural network) can predict the onset of the Indian monsoon better than other models. Machine learning uncovered connections between extreme-rainfall events occurring in different parts of the globe, and can forecast the El Niño–Southern Oscillation.
Looking backward in time
Besides forecasting, artificial intelligence has been able to successfully reconstruct missing historical climate information. Historical data are often very sparse before large-scale observations were introduced in the mid-20th century. For some observables (eg temperature, precipitation), we have observations for just a few years. Without knowledge of the past, we cannot quantify historical changes, making it even harder to predict the future.
AI models are trained on climate model output and can reconstruct the spatial and temporal extent of various real-world variables. In other words, the AI model learns from the climate model and can apply that knowledge to the real world. They have been shown to outperform other widely used methods in geosciences. However, by the nature of the problem, it is hard to ensure and verify that the results are plausible because we cannot compare our reconstruction with the observation, since that is what we want to reconstruct. We can, however, compare our reconstructions with known historical events.
Several historical events led to droughts, bad harvests, and famines. One example is the warm phase of the El Niño–Southern Oscillation, which has a clear signature in the surface temperatures in the Pacific Ocean. During the years 1876–1877, the El Niño led to the most deadly famines during the 19th century.
However, we only have very sparse observations of the surface temperature during that time. While widely used statistical methods are not able to reconstruct the spatial patterns of the El Niño in 1876/77, a reconstruction with machine learning models reveals the extraordinarily warm Pacific during that time.
Saving supercomputer time
There is also an active effort to leverage and combine the advantages of machine learning with classical Earth system models. These climate models are computationally extremely expensive and often cannot be run further than a few centuries into the future, and even then only with low resolutions of several hundred kilometres.
A single simulation with a state-of-the-art climate model for a century can take several weeks or months of wall time on a supercomputer. However, we often need ensembles of simulations – a huge number of simulations from the same model – to do statistical analyses and ensure that we do not, by chance, get a simulation at the extreme end of all possible outcomes. Climate models show what we call internal variability, which is the naturally occurring variation on different timescales and the chaotic nature of the Earth system.
While AI models often need exceedingly time-consuming fine-tuning and training, they can generate results several magnitudes faster than classical models once they are trained. This allows us, in principle, to run large ensemble simulations with higher resolutions.
As of today, we have the first prototypes of hybrid Earth system models, and the first validated models are expected within the next few years.
Plausibility and “explainability”
One of the biggest questions that the application of artificial intelligence poses in climate science is the physical plausibility of these models. How can we ensure that the results make sense in a physical way?
Classical numerical models are governed by the laws of physics, often differential equations that conserve quantities such as energy or momentum. We can, in theory, make sense of the results and explain them in a physical manner. However, machine learning models are often not physically restrained or explainable.
For example, diffusion models have problems with generating human hands. They often dream of more than five fingers if we ask them to generate a human hand. While it is easy for us to see seven fingers as unconvincing, implausibility is not so obvious in complex physical systems such as the climate system of the Earth.
Recent efforts try to include physical constraints into machine learning to achieve what are called physics-informed neural networks. There, the model is penalised for generating “unphysical results”. However, physically constraining a model is often not trivial or feasible. The “explainability” of AI models is another issue. Often we do not know how the models achieve the results. Especially when the results are unexpected, it is important to understand why the model behaves the way it does. In the context of weather and climate, we need trustable results that we can communicate with certainty to the public and policymakers, and that is why explainability is so important.
Saving scientists’ time?
In addition to its direct application in research, AI tools can also boost the productivity of scientists. For example, tools like ChatGPT and GitHub Copilot can be surprisingly effective in sketching and improving code, provided that the instructions are clear. Researchers, who often teach themselves programming, can particularly benefit from such tools. These models can improve the quality of their code and save them time that could be better spent on other tasks.
AI models are capable of comprehending long articles when busy scientists do not have enough time to read them, and they can even formulate emails when we struggle to find the right words. But why are these models so effective? Often, they are trained on massive datasets – tens or even hundreds of terabytes of data – and it is not always clear whether the copyrights of the owners are being respected. This issue is often ignored but should be kept in mind.
Furthermore, these models can give wrong answers or results that can only be identified as incorrect by people with expert knowledge. Trusting the model output blindly can significantly harm the scientific community and the public. There have been several cases of AI-generated content in scientific articles.
In the best-case scenario, AI can lead to no or only minor inaccuracies, while in the worst-case scenario, it can result in false statements in scientific articles based on faulty and unaudited output from AI models.
Therefore, most publishers prohibit the use of large language models for writing scientific articles, and rightly so.
Outlook
While AI can be an incredibly valuable tool for researchers, enhancing our understanding of the Earth and increasing productivity, it is not a panacea. Careless use of AI can have negative consequences for both science and society.
Further reading
-
Fiskeri- og havbruksvitenskap - bachelor
Varighet: 3 År -
Fiskeri- og havbruksvitenskap - master
Varighet: 2 År -
Akvamedisin - master
Varighet: 5 År -
Bioteknologi - bachelor
Varighet: 3 År -
Arkeologi - master
Varighet: 2 År -
Musikkteknologi
Varighet: 2 Semestre -
Computer Science - master
Varighet: 2 År -
Geosciences - master
Varighet: 2 År -
Biology - master
Varighet: 2 År -
Technology and Safety in the High North - master
Varighet: 2 År -
Physics - master
Varighet: 2 År -
Mathematical Sciences - master
Varighet: 2 År -
Biomedicine - master
Varighet: 2 År -
Molecular Sciences - master
Varighet: 2 År -
Biologi - bachelor
Varighet: 3 År -
Medisin profesjonsstudium
Varighet: 6 År -
Luftfartsfag - bachelor
Varighet: 3 År -
Arkeologi - bachelor
Varighet: 3 År -
Informatikk, datamaskinsystemer - bachelor
Varighet: 3 År -
Informatikk, sivilingeniør - master
Varighet: 5 År -
Geologi - bachelor
Varighet: 3 År -
Biomedisin - bachelor
Varighet: 3 År -
Kjemi - bachelor
Varighet: 3 År -
Samfunnssikkerhet og miljø - bachelor
Varighet: 3 År -
Matematikk - årsstudium
Varighet: 1 År -
Ergoterapi - bachelor
Varighet: 3 År -
Fysioterapi - bachelor
Varighet: 3 År -
Radiografi - bachelor
Varighet: 3 År -
Automasjon, ingeniør - bachelor (ordinær, y-vei)
Varighet: 3 År -
Samfunnssikkerhet - master
Varighet: 2 År -
Farmasi - bachelor
Varighet: 3 År -
Farmasi - master
Varighet: 2 År -
Romfysikk, sivilingeniør - master
Varighet: 5 År -
Klima og miljøovervåkning, sivilingeniør - master
Varighet: 5 År -
Bærekraftig teknologi, ingeniør - bachelor
Varighet: 3 År -
Forkurs for ingeniør- og sivilingeniørutdanning
Varighet: 1 År -
Odontologi - master
Varighet: 5 År -
Anvendt fysikk og matematikk, sivilingeniør - master
Varighet: 5 År -
Praktisk-pedagogisk utdanning for trinn 8-13 (deltid) - årsstudium
Varighet: 2 År -
Internasjonal beredskap (samlingsbasert) - bachelor
Varighet: 3 År -
Datateknikk, ingeniør - bachelor (y-vei)
Varighet: 3 År -
Droneteknologi, ingeniør - bachelor
Varighet: 3 År -
Bygg, ingeniør - bachelor
Varighet: 3 År -
Bygg, ingeniør - bachelor (nettbasert)
Varighet: 3 År -
Bygg, ingeniør - bachelor (y-vei)
Varighet: 3 År -
Datateknikk, ingeniør - bachelor
Varighet: 3 År -
Datateknikk, ingeniør - bachelor (nettbasert)
Varighet: 3 År -
Elkraftteknikk, ingeniør - bachelor
Varighet: 3 År -
Elektronikk, ingeniør - bachelor
Varighet: 3 År -
Elektronikk, ingeniør - bachelor (y-vei)
Varighet: 3 År